Secure AI: Decentralized Tech Fixes AI’s Trust Problem
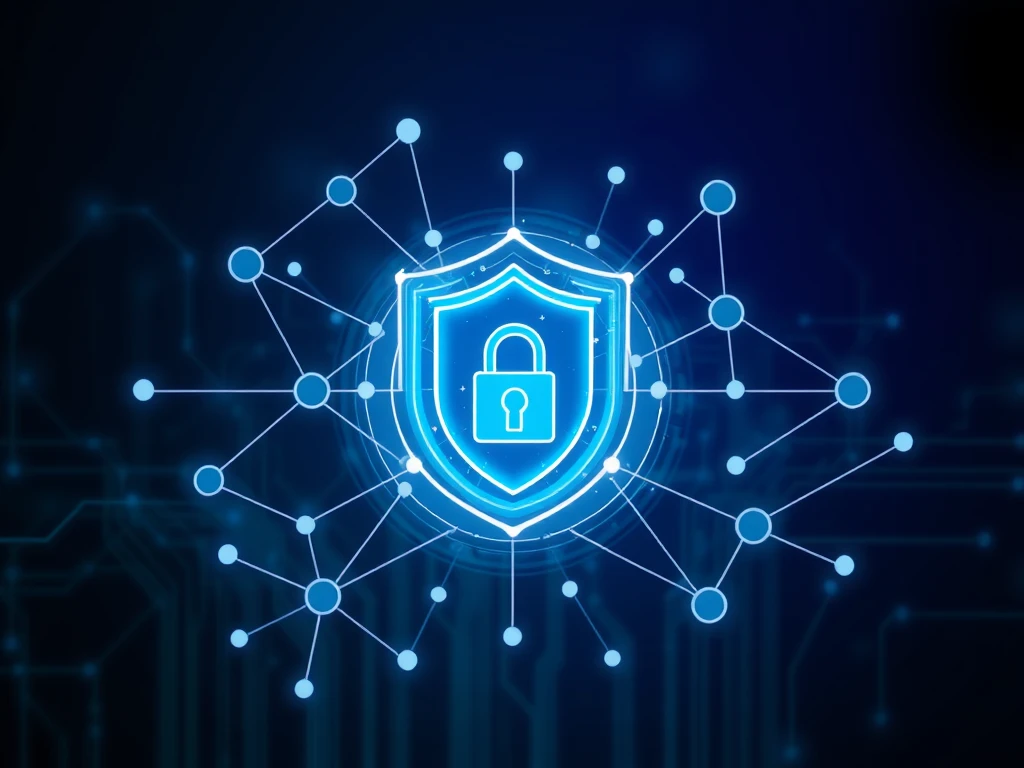
Artificial intelligence has captured significant attention in 2024 and beyond, yet a crucial hurdle remains: widespread **AI trust**. Despite massive investment and integration into finance, healthcare, and personal data management, users and companies express hesitation about AI’s reliability and integrity. This growing trust deficit poses a significant barrier to truly broad AI adoption. Fortunately, decentralized, privacy-preserving technologies are emerging as powerful solutions, offering verifiability, transparency, and robust data protection without hindering AI’s potential.
Understanding the Pervasive AI Trust Deficit
AI quickly became a dominant theme in 2024, particularly within the crypto space, attracting substantial investor interest. Startups and large corporations have channeled resources into expanding AI into critical areas like finance and health. The emerging DeFi x AI (DeFAI) sector, for example, demonstrated AI’s capacity to make decentralized finance more intuitive and powerful, enabling complex operations via simple commands and enhancing market analysis. However, innovation alone has not solved AI’s fundamental vulnerabilities:
- Hallucinations: AI generating incorrect or fabricated information.
- Manipulation: AI systems being tricked or prompted to act against their programming.
- Privacy Concerns: The risk of sensitive data being exposed or misused when processed by AI.
A notable incident in November 2024, where a user manipulated an AI agent on Base to transfer $47,000 despite its safety protocols, highlighted the real risks of trusting AI with autonomous financial operations. While audits and bug bounties help, they don’t eliminate risks like prompt injection or unauthorized data use.
Hesitation is widespread. A 2023 KPMG report found 61% of people still hesitate to trust AI. Industry professionals share this concern; a Forrester survey cited in Harvard Business Review identified trust as AI’s biggest obstacle for 25% of analysts. This skepticism persists, with a Wall Street Journal poll showing 61% of top US IT leaders are still only experimenting with AI agents, citing reliability, cybersecurity, and data privacy as top concerns. Industries like healthcare face these risks acutely, where sharing electronic health records (EHR) to train AI algorithms is promising but legally and ethically challenging without strong privacy measures.
How Decentralized Privacy-Preserving Tech Builds Trust
Building trust is not optional for AI; it’s essential for realizing its projected economic impact. This is where decentralized cryptographic systems, such as **ZK-SNARKs** (Zero-Knowledge Succinct Non-Interactive Arguments of Knowledge), offer a new path. These technologies allow users to verify AI decisions and outputs without revealing the underlying personal data or the AI model’s proprietary logic. By applying privacy-preserving cryptography to machine learning infrastructure, AI systems can become auditable, trustworthy, and respectful of privacy, which is critical for sensitive sectors like finance and healthcare.
**ZK-SNARKs** utilize advanced cryptographic proofs that enable one party to prove a statement is true to another without disclosing the specific information used to prove it. In the context of AI, this means:
- AI models can be verified for correct operation without exposing their training data or internal architecture.
- Inputs to AI models can be proven to meet certain criteria (e.g., a credit score threshold) without revealing the actual input value.
- AI outputs can be verified for integrity and correctness without revealing the model’s internal logic or sensitive data processed.
Imagine a decentralized AI lending application. Instead of accessing full financial records, it could use ZK-SNARKs to verify encrypted proofs of creditworthiness, enabling autonomous loan decisions while protecting user privacy and institutional risk. This technology also helps address the ‘black-box’ problem of large language models (LLMs), allowing verification of outputs while shielding both data integrity and model intellectual property.
The Rise of Decentralized AI and Blockchain Integration
We are entering a new phase where simply having better AI models is insufficient. Users demand transparency, enterprises require resilience, and regulators expect accountability. Decentralized, verifiable cryptography provides these elements. Technologies like **ZK-SNARKs**, threshold multiparty computation (MPC), and BLS-based verification systems are not just niche ‘crypto tools’; they are becoming foundational components for building trustworthy AI systems.
Combined with the inherent transparency and immutability of blockchain technology, these privacy-preserving methods create a powerful new infrastructure stack for AI. This stack enables systems that are not only privacy-respecting but also auditable and reliable. This synergy between decentralized technologies and AI is paving the way for truly trustworthy **blockchain AI** applications.
Gartner predicted that 80% of companies would use AI by 2026. Achieving this widespread adoption won’t be driven solely by hype or resources. It will fundamentally depend on building AI systems that people and companies can genuinely trust. And achieving that level of trust starts with embracing decentralized and privacy-preserving technologies.
Opinion by: Felix Xu, co-founder of ARPA Network and Bella Protocol. This article is for general information purposes and is not intended to be and should not be taken as legal or investment advice. The views, thoughts, and opinions expressed here are the author’s alone and do not necessarily reflect or represent the views and opinions of Crypto News Insights.