SUCCESS: Decentralized AI Data from OORT Hits Top Ranks on Google Kaggle
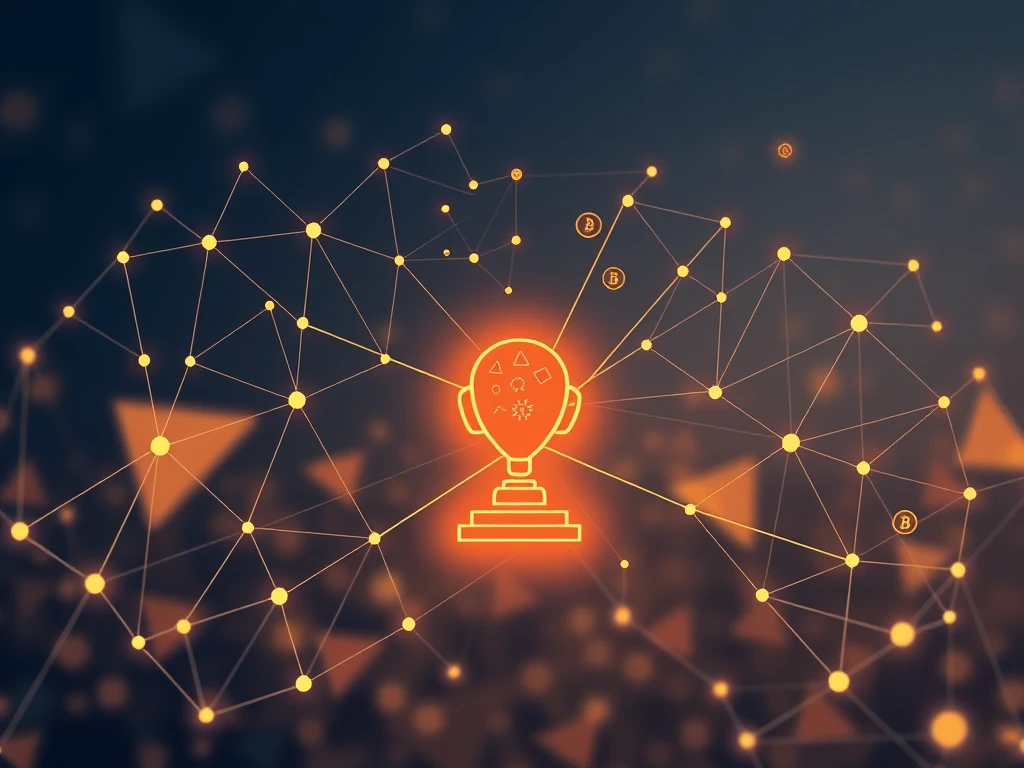
The world of artificial intelligence is hungry for high-quality data, and a recent achievement by decentralized AI solution provider OORT on Google’s data science platform, Kaggle, is turning heads. For those in the crypto space, this isn’t just a tech story; it’s a validation of how decentralized models can tackle real-world data challenges and gain significant traction.
OORT AI Data Achieves Google Kaggle Success
OORT’s AI image data set, specifically the ‘Diverse Tools’ listing, made a notable splash on Google Kaggle. Released in early April, the dataset quickly climbed to the first page in several key categories. Google Kaggle is a prominent online platform for data science and machine learning professionals, hosting competitions, datasets, and collaboration tools.
Ramkumar Subramaniam, a core contributor at crypto AI project OpenLedger, commented on this achievement, stating that a front-page Kaggle ranking serves as a strong social signal. It indicates that the dataset is effectively reaching and engaging the relevant communities of data scientists, machine learning engineers, and practitioners.
Why Decentralized AI Data Matters
Max Li, founder and CEO of OORT, highlighted the significance of the engagement metrics observed on Kaggle. He sees this as validation for the early demand and relevance of OORT’s training data, which is gathered through a decentralized model. Li explained:
The organic interest from the community, including active usage and contributions — demonstrates how decentralized, community-driven data pipelines like OORT’s can achieve rapid distribution and engagement without relying on centralized intermediaries.
This success suggests that a decentralized approach can build robust data pipelines that resonate with the data science community.
The Scarcity of Quality AI Training Data
Reports have circulated for years about the increasing scarcity of high-quality AI training data, particularly human-generated content. AI research firm Epoch AI estimates that human text data could be exhausted as early as 2028. This pressure is driving significant investment and even deals to secure rights to copyrighted materials.
While synthetic (AI-generated) data is increasingly used, human data is still largely considered superior for training better AI models. The situation is further complicated in the image data space by techniques like ‘poisoning,’ where artists intentionally alter images to degrade AI model performance, aiming to protect their work from unauthorized use.
Subramaniam pointed out the dual challenge facing open-source datasets in this environment:
- Quantity: Simply having enough data.
- Trust: Verifying the data’s provenance and integrity, especially with adversarial techniques like image poisoning on the rise.
OORT AI’s Approach: Provenance and Incentives
While a Kaggle ranking is an achievement, Subramaniam cautioned it’s not the sole indicator of real-world enterprise-grade quality. He emphasized that what truly sets OORT’s data set apart is its ‘provenance and incentive layer.’ Unlike centralized vendors with potentially opaque processes, OORT’s system is designed to be transparent and uses token incentives. This offers:
- Traceability: Knowing where the data comes from.
- Community Curation: Allowing the community to help maintain data quality.
- Potential for Continuous Improvement: Assuming proper governance is in place.
Lex Sokolin, a partner at AI venture capital firm Generative Ventures, noted that while replicating such results might not be impossible, OORT’s success does demonstrate that crypto projects can effectively use decentralized incentives to organize economically valuable activities.
What’s Next for OORT and Decentralized AI?
Max Li confirmed that OORT plans to release several other datasets in the coming months. These include specialized datasets like:
- In-car voice commands
- Smart home voice commands
- Deepfake videos (for improving AI media verification)
In an era where high-quality data is becoming scarce and trust is paramount due to techniques like image cloaking, verifiable and community-sourced incentivized datasets like OORT’s are becoming increasingly valuable. Subramaniam believes such projects can evolve from being mere alternatives to becoming fundamental pillars for AI alignment and provenance within the data economy.
Conclusion
OORT’s success on Google Kaggle is more than just a platform ranking; it’s a significant signal validating the potential of decentralized AI data solutions. In a data landscape facing scarcity and integrity challenges, OORT’s community-driven, token-incentivized approach offers a promising model for sourcing, curating, and distributing the high-quality data needed to train the next generation of AI models. This achievement underscores the growing intersection of crypto, decentralization, and the burgeoning field of artificial intelligence, demonstrating tangible outcomes for Web3 initiatives in addressing critical industry needs.